Summary
Classifies LAS points from overlapping scans of aerial lidar surveys.
Illustration
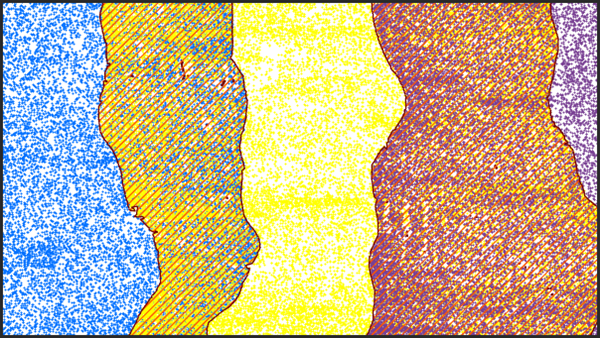
Usage
Unclassified overlap points may produce undesirable results in operations that assume regular point distribution, such as the Classify LAS Building tool. Data derived from such points may also have an undesirable margin of error from returns with the larger magnitude scan angles. Classifying overlap points allows them to be excluded to achieve a more even point distribution of higher quality returns.
This tool processes the input LAS data on a file-by-file basis and, consequently, requires the LAS files to have no overlapping coverage. If the LAS files have overlapping coverage, they can be merged into nonoverlapping files using the Tile LAS tool.
The point source ID attribute of a LAS point provides information about the flight line from which it was collected. This tool processes LAS data in tiles by determining if multiple point source IDs are present, then identifying the ID with the higher magnitude scan angle as the overlap. If multiple points with the same point source ID exist in the area being processed, all the points that share the point source ID of the point with the largest magnitude scan angle will be classified as overlap. For this reason, the sample size used to evaluate the LAS points should be approximately two to three times the size of the nominal point spacing of the LAS data. Larger tile sizes should be avoided because it risks the possibility of misclassifying points with smaller scan angle values. Smaller sample sizes may not capture enough points to properly identify and classify any overlap points.
Overlap points in LAS version 1.4 files and point record formats 6 through 8 will be assigned the overlap classification flag while retaining their original class code value. Overlap points in all other supported LAS files will be assigned a class code value of 12. If the class code value of 12 is already being used by the input LAS files to represent something other than overlapping scans, consider using the Change LAS Class Codes tool to reassign those points to another value prior to executing this tool.
Syntax
ClassifyLasOverlap(in_las_dataset, sample_distance, {extent}, {process_entire_files}, {compute_stats})
Parameter | Explanation | Data Type |
in_las_dataset | The tiled LAS dataset to process. | LAS Dataset Layer |
sample_distance | The distance of either dimension of the square area used to evaluate the LAS data. This value can be expressed as a number and a linear unit value, such as 3 meters. If linear units are not specified or are entered as Unknown, the unit will be defined by the input LAS file's spatial reference. | Linear Unit |
extent (Optional) | Specifies the extent of the data that will be evaluated by this tool. | Extent |
process_entire_files (Optional) | Specifies how the processing extent is applied.
| Boolean |
compute_stats (Optional) | Specifies whether statistics should be computed for the LAS files referenced by the LAS dataset. Computing statistics provides a spatial index for each LAS file, which improves analysis and display performance. Statistics also enhance the filtering and symbology experience by limiting the display of LAS attributes, like classification codes and return information, to values that are present in the LAS file.
| Boolean |
Derived Output
Name | Explanation | Data Type |
out_las_dataset | The LAS dataset to be modified. | LAS Dataset Layer |
Code sample
ClassifyLasOverlap example 1 (Python window)
The following sample demonstrates the use of this tool in the Python window.
arcpy.env.workspace = 'C:/data'
arcpy.ddd.ClassifyLasOverlap('Denver_2.lasd', '1 Meter')
ClassifyLasOverlap example 2 (stand-alone script)
The following sample demonstrates the use of this tool in a stand-alone Python script.
'''****************************************************************************
Name: Classify Lidar & Extract Building Footprints
Description: Extract footprint from lidar points classified as buildings,
regularize its geometry, and calculate the building height.
****************************************************************************'''
import arcpy
lasd = arcpy.GetParameterAsText(0)
dem = arcpy.GetParameterAsText(1)
footprint = arcpy.GetParameterAsText(2)
try:
desc = arcpy.Describe(lasd)
if desc.spatialReference.linearUnitName in ['Foot_US', 'Foot']:
unit = 'Feet'
else:
unit = 'Meters'
ptSpacing = desc.pointSpacing * 2.25
sampling = '{0} {1}'.format(ptSpacing, unit)
# Classify overlap points
arcpy.ddd.ClassifyLASOverlap(lasd, sampling)
# Classify ground points
arcpy.ddd.ClassifyLasGround(lasd)
# Filter for ground points
arcpy.management.MakeLasDatasetLayer(lasd, 'ground', class_code=[2])
# Generate DEM
arcpy.conversion.LasDatasetToRaster('ground', dem, 'ELEVATION',
'BINNING NEAREST NATURAL_NEIGHBOR',
sampling_type='CELLSIZE',
sampling_value=desc.pointSpacing)
# Classify noise points
arcpy.ddd.ClassifyLasNoise(lasd, method='ISOLATION', edit_las='CLASSIFY',
withheld='WITHHELD', ground=dem,
low_z='-2 feet', high_z='300 feet',
max_neighbors=ptSpacing, step_width=ptSpacing,
step_height='10 feet')
# Classify buildings
arcpy.ddd.ClassifyLasBuilding(lasd, '7.5 feet', '80 Square Feet')
#Classify vegetation
arcpy.ddd.ClassifyLasByHeight(lasd, 'GROUND', [8, 20, 55],
compute_stats='COMPUTE_STATS')
# Filter LAS dataset for building points
lasd_layer = 'building points'
arcpy.management.MakeLasDatasetLayer(lasd, lasd_layer, class_code=[6])
# Export raster from lidar using only building points
temp_raster = 'in_memory/bldg_raster'
arcpy.management.LasPointStatsAsRaster(lasd_layer, temp_raster,
'PREDOMINANT_CLASS', 'CELLSIZE', 2.5)
# Convert building raster to polygon
temp_footprint = 'in_memory/footprint'
arcpy.conversion.RasterToPolygon(temp_raster, temp_footprint)
# Regularize building footprints
arcpy.ddd.RegularizeBuildingFootprint(temp_footprint, footprint,
method='RIGHT_ANGLES')
except arcpy.ExecuteError:
print(arcpy.GetMessages())
Environments
Licensing information
- Basic: Requires 3D Analyst
- Standard: Requires 3D Analyst
- Advanced: Requires 3D Analyst