Workflow using ArcGIS Pro
This tutorial covers key components of the political analyst's workflow. It reviews what she did to download and prepare the data, then provides step-by-step instructions for examining broad spatial correlations at a national level and finding properly specified regression models for a three-state region (Arizona, Colorado, and New Mexico). While in this example the methods are used to analyze election results, you can apply these methods in many other applications where you want to explore the relationships between variables.
Collect the data
The analyst's initial dataset included every county in the continental United States.
Using tools such as Enrich Layer, Add Field, Calculate Field, and Add Join the analyst added the 2016 presidential vote percentages (Republican and Democratic) to the counties layer attribute table, along with about 30 socioeconomic explanatory variables.
Later, for the regional analysis, she used the same tools to add a number of additional variables, including Tapestry LifeMode and Urbanization categories. She also used the Near tool to calculate the distance each county in the region lies from the United States - Mexico border and from a major city.
The downloadable dataset includes layers containing fewer explanatory variables for both the national analysis and the Southwestern regional analysis, to speed up the processing as you work through the analysis.
Since your analysis will be composed of fewer explanatory variables, the reports you generate will look slightly different than the ones the analyst created. However, your results will be essentially the same as the analyst's.
Determine which explanatory variables are consistent predictors of the percentage Trump vote
To get a feeling for which variables were consistently good predictors of Trump support, the political analyst used Exploratory Regression. Using Exploratory Regression with a number of relevant explanatory variables is a quick way to see which variables you've collected are consistently good predictors of another variable (the dependent variable). You can complete the steps below using the provided data.
- Run Exploratory Regression with these parameters.
- Input features: CountyData_EnrichLayer
- Dependent Variable: PCT_GOP
- Candidate Explanatory Variables:
- 2016 Ave HH Income
- 2016 Diversity Index
- 2016 Population Density
- % Seniors (Age 65+)
- % Republican
- % Blue Collar Workers
- % Never Married
- % College Degree
- Output Report File: CountyData_ERNational.txt (or another name of your choosing)
- Navigate to the folder containing the report and open the report.
Since at this point you are only trying to identify significant variables, and not looking for properly specified models, you can run Exploratory Regression with the default parameters.
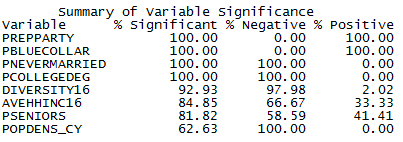
Analyze correlations and how they change across the nation
- Run Geographically Weighted Regression (GWR) using the percent Trump vote as the dependent variable and the percent blue collar workers as the only explanatory variable. Each county will get a coefficient value indicating how strong the correlation between these two variables is.
- Input features: CountyData_EnrichLayer
- Dependent variable: PCT_GOP
- Explanatory variable: % Blue Collar Workers
- Output feature class: GWR_BlueCollar
- Kernel type: Fixed
- Bandwidth method: Akaike Information Criterion
- Examine the output feature class.
- GWR creates an output feature class of counties with a number of fields. The default map displays the values for the standard residuals field (Std. Residuals). However, the field of interest for this analysis is the one labeled Coefficient #1 pBlueCollar (the field will always be labeled Coefficient #1, followed by the name of the explanatory variable). Open the layer's attribute table to view the field.
- A positive value for the coefficient indicates a positive relationship between the explanatory variable and the dependent variable—as the percentage of blue collar workers in a county increases, the percent GOP vote also increases.
- The absolute magnitude of the coefficient indicates the strength of the relationship. For example, for % Blue Collar Workers, a coefficient of 3.0 for a county means that a one percent increase in the percentage of blue collar workers is associated with a three percent increase in the GOP vote. .
- To see the pattern of where the relationship is strongest, map the coefficient field (for example, Coefficient #1 pBlueCollar) using graduated colors.
- Run GWR again using % College Degree as the explanatory variable, or experiment with some of the other available variables, and examine the output feature class.
- For % College Degree, the relationship is primarily negative—as the percentage of people with a college degree in a county increases, the percent GOP vote decreases. A coefficient of -1.5 indicates that a one percent increase in the percentage of people with a college degree in a county results in a decrease of 1.5 percent in the GOP vote.
Add explanatory variables and Identify battleground counties
- In Fields View, add a new field to the CountyData_EnrichLayer layer. Name the field pGOP_minus_pDEM, and assign a Data Type of Float.
- Use Calculate Field to calculate the values for the new field by subtracting the percentage 2016 Democratic vote (PCT_DEM) from the percentage 2016 GOP (PCT_GOP) vote.
- Use Select Layer by Attribute to select the counties where the pGOP_minus_pDEM value is between -0.2 and 0.2.
- Use Copy Features to copy the selected counties to a new data layer.
- Using the new layer, create the map of battleground counties using Graduated Colors and specifying the pGOP_minus_pDEM field with a Manual Interval and two categories: values between 0 and 0.2 (an Upper value of 0.2) were counties that Trump won by less than 20 percent; values between 0 and -0.2 (an Upper value of 0.0) were counties that Clinton won by less than 20 percent. Select a red color for the former and a blue color for the latter, and update the labels.
To prepare for finding a properly specified model for counties at a state or regional level the analyst added more explanatory variables to the counties layer, including several distance variables to account for spatial trends or underlying spatial processes associated with Trump voting patterns. The chances of finding a properly specified model increase with more explanatory variables—as long as they are relevant and justifiable. She then identified battleground counties to focus her analysis.
Once the analyst had added the additional explanatory variables, she set about finding and selecting a subset of counties for her regional analysis. You can perform these steps with the continental United States counties layer.
Modeling the regional vote
- Run Exploratory Regression using the following parameters.
- Input Features: AZ_CO_NM_EnrichLayer
- Dependent Variable: PCT_GOP
- Candidate Explanatory Variables:
- 2016 Ave HH Income
- 2016 Total Population
- Median Age
- % Republican
- per HH Cash Contributions to Churches/Rel Org
- % White
- % Hispanic
- % HH w Children
- % Savvy Suburbs 1D
- % RustBelt 5D
- % Southern Sat 10A
- % Rooted Rural 10B
- % College Degree
- MedYearMovedIn
- Output Report File: AZ_CO_NM_ER3vars.txt (or a name of your choosing)
- Under Search Criteria specify the following (models that meet these criteria are considered properly specified, and deemed "passing" models).
- Maximum Number of Explanatory Variables: 3
- Minimum Number of Explanatory Variables: 1
- Minimum Acceptable Adj R Squared: 0.8 (model must explain at least 80% of variation in the vote totals)
- Maximum Coefficient p value Cutoff: 0.01 (there must be 99% certainty that any variables in a given model were not included due to chance)
- Maximum VIF Value Cutoff: 5 (reduces the acceptable level of redundancy between variables, termed multicollinearity)
- Minimum Acceptable Jarque Bera p value: 0.1 (default)
- Minimum Acceptable Spatial Autocorrelation p value: 0.1 (default)
- Examine the report created by Exploratory Regression to see if there are any passing models (the report should show that there aren't any) and to identify poorly correlated variables.
The Exploratory Regression report using one, two, and three variable models. While the best-scoring variables are shown, none of the combinations meet the analyst's stringent criteria for a passing model (the Passing Models header appears, but no models are listed, as indicated by the circled areas). Exploratory Regression runs Ordinary Least Squares (OLS) regression for every combination of candidate explanatory variables up to the number of variables you specify to include in the model (so if you specify that models include three variables, Exploratory Regression tries every possible combination of variables, three at a time). This can take time to process, especially for models with five or more variables. To speed up the processing, examine the report created by Exploratory Regression to look for variables that can be excluded from the analysis. (While not required with the small number of variables included here, these techniques are useful if you have many features and many candidate explanatory variables. Excluding poorly correlated variables is an effective strategy to speed up the Exploratory Regression process.)
- In the Summary of Mutlicollinearity section of the report, identify for exclusion any variables having high multicollinearity, say over 85% or 90%. These are variables that are redundant with one or more other variables. Having redundancy in a model can make the results more suspect.
Two variables—AVEHHINC16 (average household income) and PHHCCCHURCHREL (percent of households contributing to churches or religious organizations)—are redundant with each other. Locate the variables in the Summary of Variable Significance section. Note the variable that is significant in more models—the other variable(s) exhibiting high multicollinearity with this variable can be excluded.
The variable that is significant in more models—AVEHHINC16—can be kept for the analysis while the other (PHHCCCHURCHREL) is excluded. - Also in the Summary of Variable Significance section identify any variables that are significant in less than 1% of the models tested. This means they are not likely factors in predicting the dependent variable. They appear at the bottom of the Summary of Variable Significance.
Some of the variables show up as significant in less than 1% of the models tested, so can be removed from the analysis.
- In the Summary of Mutlicollinearity section of the report, identify for exclusion any variables having high multicollinearity, say over 85% or 90%. These are variables that are redundant with one or more other variables. Having redundancy in a model can make the results more suspect.
- Exclude these poor performers from the next run of Exploratory Regression by unchecking them in the Visible column in Fields View in the AZ_CO_NM_EnrichLayer layer's attribute table. That way, they won't appear in the Exploratory Regression dialog. (Alternatively, you can uncheck the variables in the Exploratory Regression dialog. However, it's much easier to keep track of what you're doing in Fields View. Plus, if you need to run Exploratory Regression again with the same data layer at a later time, the variables will still be turned off and you won't have to uncheck them again in the dialog.) Uncheck the Visible checkbox for the variables below, and click Save.
- per HH Cash Contributions to Churches/Rel Org
- % Rooted Rural 10B
- % Savvy Suburbs 1D
- 2016 Total Population
- % RustBelt 5D
- % Southern Sat 10A
- Run Exploratory Regression using 4 as the Maximum and Minimum number of explanatory variables. Use the same parameters as before, but include the remaining eight explanatory variables from the original list, and change the name of the report to AZ_CO_NM_ER4vars.txt. Examine the report to see if there are any passing models.
- Repeat Step 4, using 5 for the Maximum and Minimum number of explanatory variables and changing the report name to AZ_CO_NM_ER5vars.txt.
- In the AZ_CO_NM_EnrichLayer attribute table, in the Visible column in Fields View, click the checkbox next to % Identifying American Indian and Change in % Hispanic 2010 to 2016. Since you are adding new variables to the analysis you should also include any variables that were excluded previously because they were significant in less than 1% of models tested. There is a chance that in combination with the new variables they will now be significant in more models—and perhaps even appear in a passing model. So in the Visible column check the boxes for the five variables you previously unchecked (see above). When you have checked all the necessary boxes, click Save.
- Re-run Exploratory Regression using the same parameters as in Step 5 above (again specifying 5 as the Maximum and Minimum number of explanatory variables). There should be a total of 15 candidate explanatory variables: the two new variables; the eight variables from the previous run; and the five variables that were excluded earlier as being significant in less than 1% of models tested. You can use the same name for the report file and overwrite the existing file. When you examine the report, it should show one passing model. Note that some variables have a positive relationship with the Trump vote while others have a negative relationship.
- Run Exploratory Regression, specifying 6 for the Maximum and Minimum number of explanatory variables, and changing the name of the report to AZ_CO_NM_ER6vars.txt. There should be three passing models. The analyst did this to find additional passing models in order to identify explanatory variables that occur in several properly specified ("passing") models.
- Using the two reports with the passing models, identify the variables that occur in at least half the passing models. They are:
- % Republican (PREPPARTY)
- % White (PWHITE)
- % Hispanic (PHISPANIC)
- % HH w Children (PHHWCHILDREN)
- % College Degree (PCOLLEGEDEG)
- Change in % Hispanic 2010 to 2016 (PHISP_DELTA_2010_2016)
- Identify the model that contains all six variables and has the highest AdjR2 value—the top six-variable model.
- Run Ordinary Least Squares (OLS) to identify the two variables having the strongest relationship with the dependent variable (PCT_GOP). While Exploratory Regression shows you whether a variable has a positive or negative relationship with the dependent variable, it doesn't tell you how strong the relationship is. The report generated by OLS includes a coefficient for each explanatory variable indicating the strength of the relationship with the dependent variable.
- Input Feature Class: AZ_CO_NM_EnrichLayer
- Unique ID Field: COMBINED_FIPS
- Output Feature Class: AZ_CO_NM_EnrichLayer_OLS
- Dependent Variable: PCT_GOP
- Explanatory Variables:
- % Republican
- % White
- % Hispanic
- % HH w Children
- % College Degree
- Change in % Hispanic 2010 to 2016
- Output Report File: AZ_CO_NM_EnrichLayer_OLS.pdf (or a name of your choosing)
- Examine the report file generated by OLS. The report shows that % Change in Hispanic Population (PCTHISP_DELT) has the largest coefficient at 1.77—a positive relationship with the dependent variable—while % College Degree (PCOLLEGEDEG) has the next largest, in absolute terms, at -1.02, a negative relationship. These are variables that would likely be the most effective for use in creating campaign messages.
The analyst's goal was to find at least one properly specified model that explained the GOP vote in the area of focus, the Southwestern United States (specifically, the three-state region composed of Arizona, Colorado, and New Mexico). When you are lucky enough to find more than one properly specified model, you can look for consistency in the variables that appear. When a variable shows up in multiple passing models you have confidence it is an important predictor. You should also look at the coefficient magnitude. Large differences from zero (either positive or negative) indicate a bigger influence on the dependent variable.
In the steps below, you will generate several properly specified models for the Arizona-Colorado-New Mexico region. You will then examine the model results and select the explanatory variables that appear in multiple passing models and have the largest (absolute value) coefficients. These variables could then be used to construct potential political messaging.
At this point, since there were still no passing models the analyst looked for additional explanatory variables her analysis might be missing, and identified the change in percent Hispanic population and the percent Identifying American Indian variables. She added these to the layer of counties for the region.
These variables are included in the provided data layer, so you don't need to run Enrich Layer to add them — you simply need to make them visible in Fields View in the layer's attribute table.